The Evolution of Sentiment Analysis: From Simple Metrics to Business Intelligence
Remember when we thought analyzing customer feedback meant counting stars and thumbs up? Those days feel like watching black and white TV now. We’ve gone from basic “positive vs negative” sorting to AI systems that can detect eye-rolling through text – and somehow, we’re still just scratching the surface of what’s possible with sentiment analysis.
Here’s the thing: most businesses are doing sentiment analysis wrong. They’re using outdated tools that treat customer feedback like a binary switch – happy or sad, thumbs up or down. But human emotions don’t work that way. We’re complex creatures who can love a product while hating its packaging, or praise a service while complaining about its price.
Understanding Modern Sentiment Analysis Examples
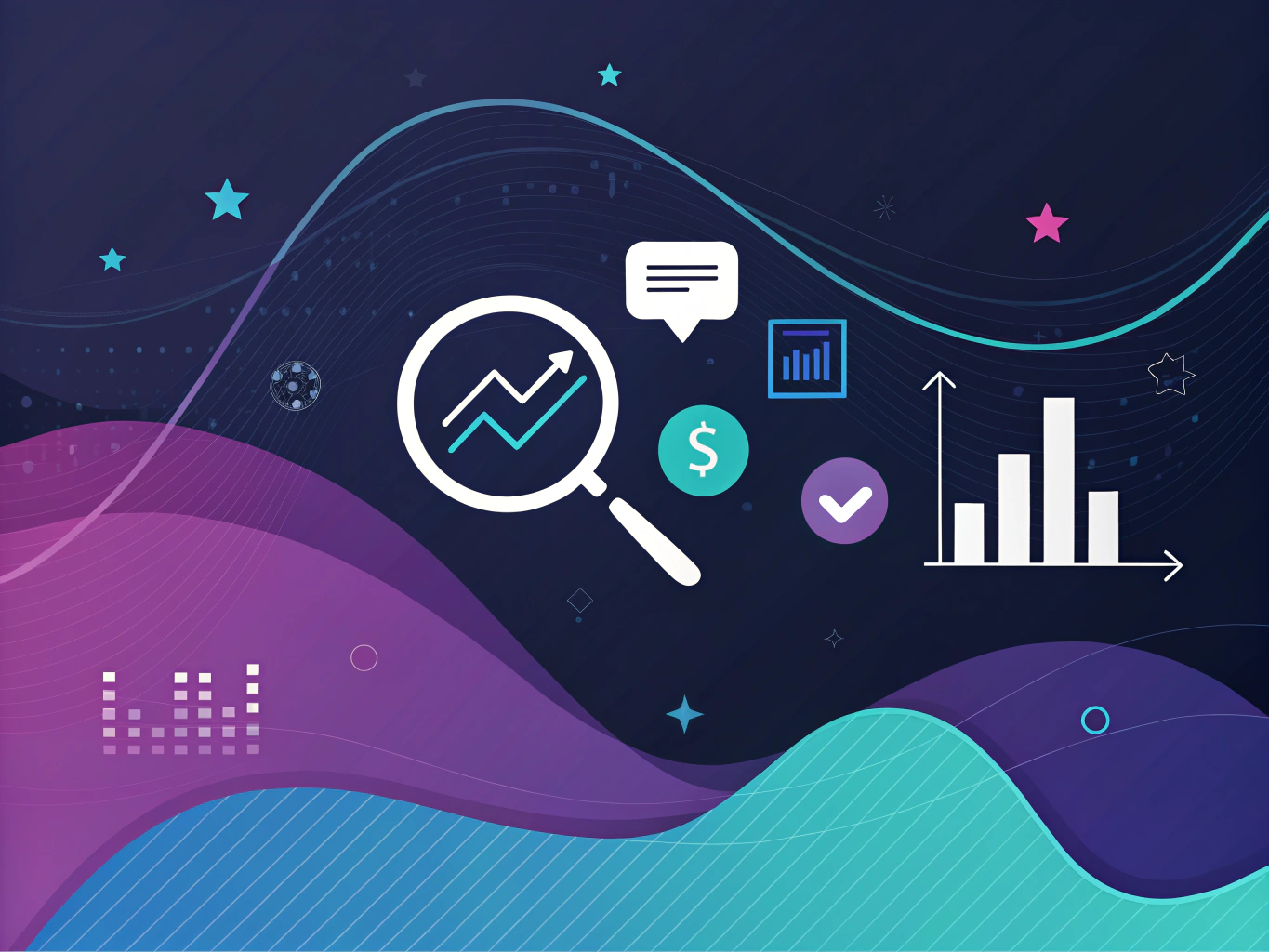
Think of sentiment analysis like having millions of tiny emotional detectives, each specialized in understanding different aspects of human communication. These digital sleuths don’t just look for obvious clues like “great” or “terrible” – they understand context, sarcasm, and even those passive-aggressive comments your customers leave when they’re trying to be “nice” about their disappointment.
The Building Blocks of Effective Sentiment Analysis
At its core, sentiment analysis is about teaching machines to understand human emotions through text. But unlike traditional NLP approaches that simply categorize words as positive or negative, modern sentiment analysis is more like an empathy engine. It considers tone, context, and cultural nuances – kind of like having a highly perceptive friend who can tell when you’re actually upset despite saying “I’m fine.”
From Basic Classification to Emotional Intelligence
The journey from basic sentiment classification to today’s sophisticated analysis tools is fascinating. We’ve moved from simple keyword spotting to understanding complex emotional states. It’s like going from a mood ring to a full psychological evaluation – except it happens in milliseconds across thousands of customer interactions simultaneously.
Real-World Applications That Actually Work
Let’s cut through the AI hype and look at how businesses are actually using sentiment analysis to drive results. Nike’s handling of the Colin Kaepernick campaign is a perfect example. They used advanced sentiment analysis to track not just the volume of social media reactions, but the underlying emotional currents driving the conversation. This allowed them to respond strategically rather than reactively to public sentiment.
Social Media Sentiment: Beyond the Basics
Social media sentiment analysis has evolved far beyond counting positive and negative mentions. Modern tools can now detect emerging trends before they become viral, identify potential PR crises in their infancy, and even predict which customer complaints are likely to escalate if left unaddressed. It’s like having a crystal ball for your brand’s social media presence – if that crystal ball was powered by machine learning and fed by millions of data points.
For instance, in real-world examples of AI topic sentiment analysis, companies have leveraged these insights to fine-tune their customer engagement strategies effectively.
Customer Support: The New Frontier
The most exciting applications of sentiment analysis are happening in customer support. Companies like Zappos aren’t just tracking customer satisfaction – they’re using sentiment analysis to identify emotional patterns that predict customer churn before it happens. Their systems can detect subtle shifts in customer sentiment across multiple interactions, allowing them to intervene before a slightly annoyed customer becomes a former customer.
Additionally, sentiment analysis is transformative for customer experiences, enabling companies to respond proactively to customer needs.
The Technical Reality Behind the Magic
While the applications of sentiment analysis can seem magical, the technology behind it is surprisingly straightforward – though not simple. Modern sentiment analysis combines several key components: natural language processing (NLP) for understanding text, machine learning for pattern recognition, and contextual analysis for understanding the bigger picture.
Breaking Down the Process
Think of sentiment analysis as a three-layer cake of understanding. The first layer is basic text analysis – identifying words and their immediate meaning. The second layer adds context – understanding how words relate to each other and what they really mean in combination. The third layer is where the magic happens – connecting individual sentiments to larger patterns and business insights.
The most effective sentiment analysis examples don’t just stop at categorizing feedback as positive or negative. They dig deeper, identifying specific aspects of products or services that drive emotional responses, tracking sentiment changes over time, and connecting customer emotions to business outcomes. It’s like having a massive focus group that never sleeps, providing constant feedback on every aspect of your business.
Real-World Sentiment Analysis Examples by Channel
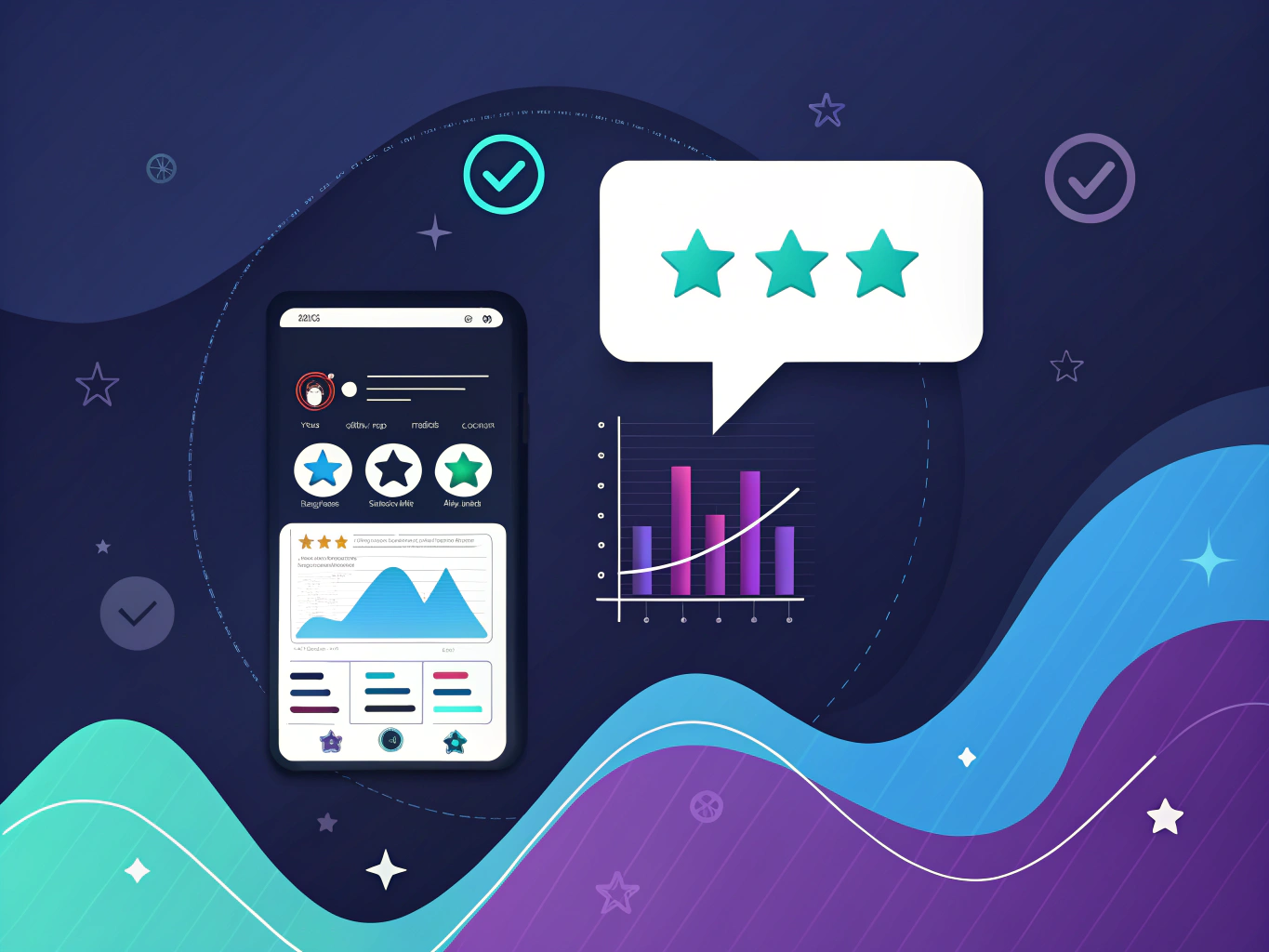
Let’s dive into the juicy stuff – actual sentiment analysis in action. Because honestly? Theory’s great, but seeing how brands are using this tech to understand their customers… that’s where it gets interesting.
Social Media Sentiment Analysis: Where the Magic (and Mayhem) Happens
Remember Nike’s Colin Kaepernick campaign? That wasn’t just a bold marketing move – it was a masterclass in sentiment analysis. Nike’s team analyzed millions of social mentions in real-time, tracking how public opinion shifted from initial shock to long-term brand loyalty. The result? A $6 billion increase in brand value, despite initial negative sentiment spikes.
Here’s what made it work: They didn’t just track positive/negative ratios. They used aspect-based sentiment analysis to understand which specific elements resonated or triggered backlash. Think of it like having thousands of focus groups running 24/7, except these people don’t know they’re being studied (creepy, but effective).
Customer Support Sentiment Analysis: Because “Fine” Usually Isn’t Fine
Take Zappos – they’re not just selling shoes, they’re selling happiness (their words, not mine). They implemented sentiment analysis across their support channels and discovered something fascinating: customers who used certain negative phrases early in their support journey were 80% more likely to return products.
The cool part? They didn’t just use this data to predict returns – they created intervention points. When their system detected these sentiment patterns, it flagged the conversation for their most experienced support staff. Return rates dropped 15% within three months. Not bad for an “intern,” right?
Survey and Feedback Sentiment Analysis: Beyond the Star Ratings
TechSmith (the Snagit folks) had a problem many SaaS companies face: tons of customer feedback, but no efficient way to process it. Their traditional survey analysis was about as effective as trying to understand a movie by reading only the one-line synopsis.
They implemented NLP sentiment analysis across their feedback channels and found that users were actually more frustrated with their documentation than their UI – something their standard satisfaction scores had missed entirely. Why? Because sentiment analysis caught the emotional undertones in comments like “Documentation is comprehensive…” (which actually meant “overwhelming and confusing” when analyzed in context).
Moreover, case studies such as using market sentiment analysis illustrate how sentiment insights can lead to more accurate and efficient business decisions.
Email Sentiment Analysis: Because Email Never Actually Died
WatchShop’s experience is particularly interesting here. They analyzed sentiment patterns in customer service emails and discovered that certain words and phrases in their automated responses were actually increasing customer frustration. It’s like they were accidentally poking the bear with perfectly polite corporate speak.
By revamping their email communication based on sentiment insights, they saw a 23% increase in positive customer sentiment scores and a 12% boost in repeat purchase rates. The key wasn’t just identifying negative sentiment – it was understanding the emotional journey throughout the entire email conversation.
Advanced Sentiment Analysis Applications
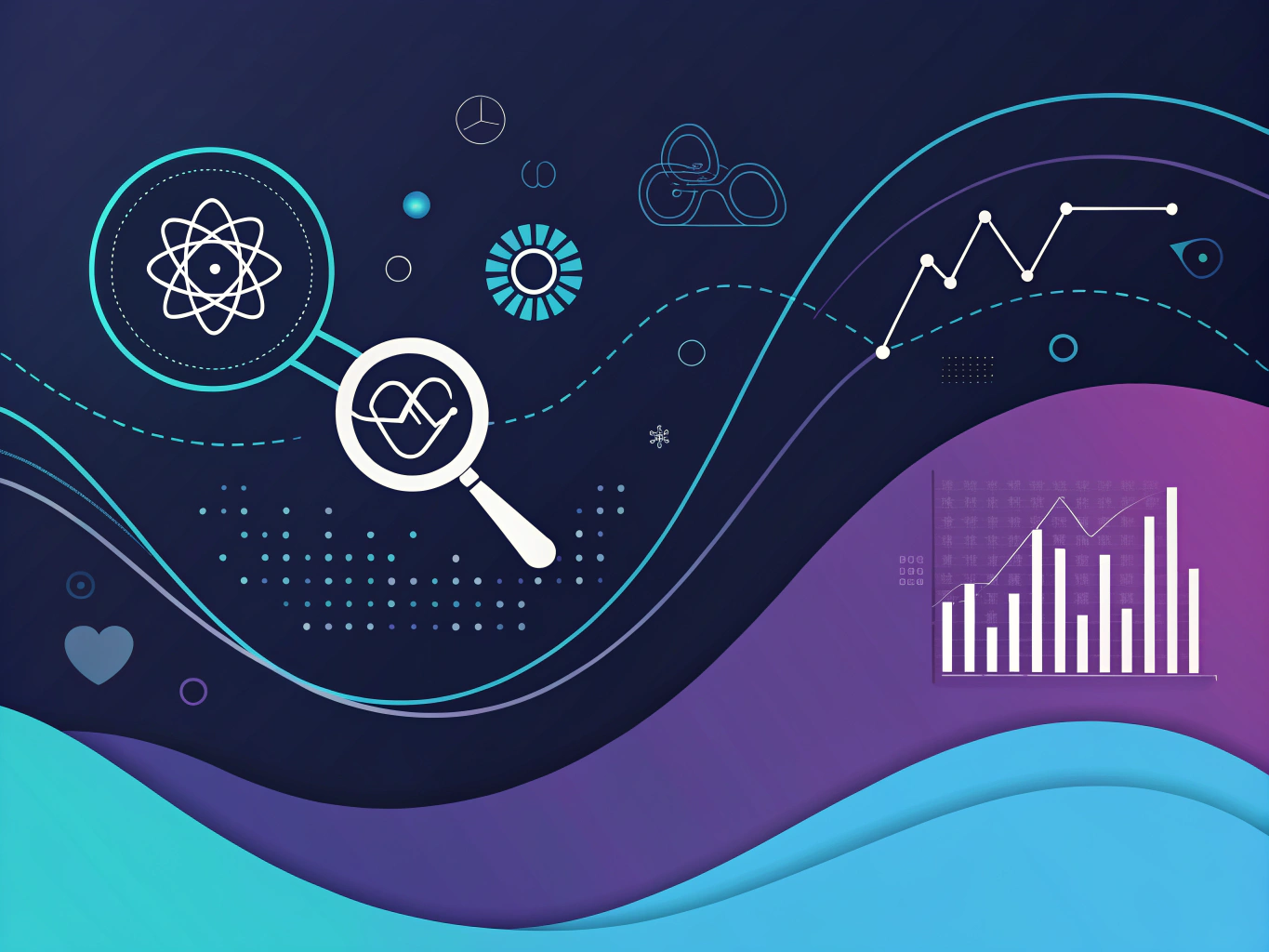
Relevance-Based Sentiment Scoring: Context is King
This is where sentiment analysis starts getting really interesting. Instead of just saying “this review is positive,” we’re now asking “how relevant is this sentiment to what we actually care about?” It’s like having a really smart filter that not only understands emotions but also knows which emotions matter for your specific business goals.
For example, when analyzing product reviews, a comment like “The packaging was terrible but the product is amazing” gets weighted differently depending on whether you’re the product manufacturer or the shipping company. Both need to know about the sentiment, but for very different reasons.
Multimodal Sentiment Analysis: Because Humans Are Complicated
Here’s where we start combining text analysis with voice tone and visual cues. Think about it – how many times have you received a “thanks” that actually meant “please go away”? Multimodal analysis catches these nuances by analyzing multiple channels simultaneously.
Contact centers are leading the charge here, combining voice tone analysis with text transcripts to identify at-risk customers before they churn. One major telecom provider reduced customer churn by 18% by identifying and proactively addressing negative sentiment patterns across channels.
Emotion Detection Beyond Positive/Negative
The binary positive/negative framework is about as nuanced as a thumb up/down – useful, but limited. Modern sentiment analysis can detect subtle emotional states like confusion, excitement, anxiety, or relief. This granularity helps brands understand the emotional journey their customers are on.
Consider how this plays out in customer journey mapping: A customer might express mild frustration early in their journey (not great, but normal), then satisfaction (good!), then confusion (uh-oh), and finally relief (phew!). Understanding these emotional transitions helps brands identify where they’re winning and where they need to improve.
Real-Time Sentiment Analysis: Because Timing is Everything
Remember the Peloton wife ad controversy? That’s a perfect example of why real-time sentiment analysis matters. What started as social media murmurs quickly snowballed into a PR crisis that wiped $1.5 billion off Peloton’s market value.
Modern sentiment analysis tools can track these sentiment shifts as they happen, allowing brands to respond before issues escalate. It’s like having an early warning system for public opinion – not perfect, but a lot better than finding out about problems through angry tweets going viral.
Real-World Sentiment Analysis Examples by Channel
Let’s dive into where sentiment analysis really shines – and trust me, it’s not just in those sterile corporate dashboards we’re all too familiar with. I’ve seen sentiment analysis transform from a nice-to-have into a must-have tool, especially for brands trying to navigate the choppy waters of social media and customer feedback.
Social Media Sentiment Analysis: Where the Magic (and Mayhem) Happens
Remember Nike’s Colin Kaepernick campaign? That wasn’t just a bold marketing move – it was a masterclass in sentiment analysis. Nike’s team tracked millions of social mentions in real-time, using tools like Brandwatch and Talkwalker to understand how the conversation was evolving. Think of it as having thousands of ears to the ground, all feeding into one central nervous system.
What’s fascinating is how they managed to separate the signal from the noise. It’s like being at a massive concert and somehow managing to hear individual conversations – except here, AI does the heavy lifting. The result? Nike could respond to genuine customer concerns while filtering out the automated noise that often floods controversial campaigns.
Customer Support Sentiment Analysis: The Front Lines of Brand Perception
Take Zappos – they’ve basically written the book on customer service, but even they needed help understanding the emotional undercurrents in their support interactions. They implemented real-time sentiment scoring in their customer interactions, which is kind of like having an emotional radar that pings when a customer’s satisfaction starts to dip.
The cool part? Their system doesn’t just flag negative sentiment – it identifies patterns. If a customer who’s usually positive suddenly turns negative across multiple interactions, that’s a red flag that gets immediate attention. It’s like having an early warning system for customer churn.
Advanced Sentiment Analysis Applications
This is where sentiment analysis starts feeling less like a basic tool and more like something out of a sci-fi novel (and as a sci-fi geek, I mean that in the best way possible).
Multimodal Sentiment Analysis: Beyond Just Words
Imagine being able to understand not just what customers are saying, but how they’re saying it. Modern sentiment analysis systems can combine text analysis with voice tone analysis and even visual cues from social media content. It’s like having a super-powered empathy machine that can read between the lines of human communication.
I recently worked with a brand that implemented this for their customer service calls. They discovered that customers who spoke in a certain tone were 80% more likely to cancel their subscription – even if their words were neutral. That’s the kind of insight that traditional analytics would miss completely.
Emotion Detection: The Next Frontier
We’re moving beyond simple positive/negative classifications into understanding the full spectrum of human emotions. Modern NLP sentiment analysis can detect joy, anger, fear, surprise – the whole emotional rainbow. It’s like upgrading from black-and-white TV to full HD color.
Implementation Strategies That Actually Work
Look, I’ve seen too many companies jump into sentiment analysis without a game plan. It’s like trying to build a house without a blueprint – you might end up with something, but it probably won’t be what you wanted.
The Right Approach for Your Business
Start small. Seriously. Pick one channel, one product line, or one customer segment. Test your sentiment analysis there before rolling it out broadly. It’s the difference between dipping your toe in the water and diving into the deep end without knowing how to swim.
For ecommerce brands, I usually recommend starting with product review sentiment analysis. It’s contained, structured, and directly tied to revenue. Plus, you can validate the results against your sales data – something that’s harder to do with social media sentiment.
Integration That Makes Sense
The best sentiment analysis implementation is one that fits naturally into your existing workflows. If your team needs to log into seven different tools to get insights, guess what? They won’t do it. Make it accessible, make it actionable, and most importantly, make it obvious how it helps them do their jobs better.
The Future of Sentiment Analysis
Here’s where things get really interesting. We’re seeing the emergence of predictive sentiment analysis – systems that can forecast how customers will feel about something before they even experience it. It’s like having a crystal ball, except it’s powered by machine learning instead of magic.
But let’s keep it real – AI isn’t going to replace human intuition in understanding emotions. What it will do is amplify our ability to understand and respond to customer feelings at scale. Think of it as having an emotional intelligence amplifier for your brand.
Final Thoughts: Making Sentiment Analysis Work for You
The key to successful sentiment analysis isn’t just in choosing the right tools or implementing the perfect algorithm. It’s about understanding that at its core, sentiment analysis is about people – their feelings, their experiences, their stories. The technology is just the lens that helps us see these things more clearly.
For brands and content creators, sentiment analysis isn’t just another analytics tool – it’s a bridge to better understanding your audience. Use it wisely, use it ethically, and most importantly, use it to create experiences that resonate with real human emotions.
Because at the end of the day, that’s what separates the brands that survive from the ones that thrive – the ability to not just hear what their customers are saying, but to truly understand how they’re feeling.
Related Articles:
- Sentiment Analysis Meaning: Decoding Customer Emotions
- Sentiment Analysis NLP: Decoding Customer Emotions
- Sentiment Analysis Dataset: Essential Tools for Beginners
Frequently Asked Questions
What is sentiment analysis with an example?
Sentiment analysis is a natural language processing technique used to determine whether data is positive, negative, or neutral. For example, a company might use sentiment analysis on social media posts to understand public opinion about a new product. If many posts contain positive words like ‘love’ and ‘great’, the sentiment is likely positive.
What is an example of a company using sentiment analysis?
Coca-Cola uses sentiment analysis to gauge consumer reactions to its marketing campaigns and product launches. By analyzing social media conversations and online reviews, they can quickly adjust their strategies based on the public sentiment to maintain a positive brand image.
How does KFC use sentiment analysis?
KFC uses sentiment analysis to monitor customer feedback across various digital platforms. This allows them to quickly identify and address customer service issues, adapt their marketing strategies, and ensure that their brand messaging resonates well with their audience.
How do you write a sentiment analysis?
Writing a sentiment analysis involves collecting data, such as text from reviews or social media, and using a sentiment analysis tool or algorithm to classify the text as positive, negative, or neutral. The results are then interpreted to understand the overall sentiment and make informed decisions based on the insights gathered.
What is sentiment analysis for beginners?
Sentiment analysis for beginners involves understanding the basic concept of analyzing text data to determine its sentiment. Beginners can start by exploring simple tools or software that automatically categorize text data, allowing them to learn how sentiment analysis can be applied in real-world scenarios, such as customer feedback or market research.
About the Author
Vijay Jacob is the founder and chief contributing writer for ProductScope AI focused on storytelling in AI and tech. You can follow him on X and LinkedIn, and ProductScope AI on X and on LinkedIn.
We’re also building a powerful AI Studio for Brands & Creators to sell smarter and faster with AI. With PS Studio you can generate AI Images, AI Videos, Blog Post Generator and Automate repeat writing with AI Agents that can produce content in your voice and tone all in one place. If you sell on Amazon you can even optimize your Amazon Product Listings or get unique customer insights with PS Optimize.
🎁 Limited time Bonus: I put together an exclusive welcome gift called the “Formula,” which includes all of my free checklists (from SEO to Image Design to content creation at scale), including the top AI agents, and ways to scale your brand & content strategy today. Sign up free to get 200 PS Studio credits on us, and as a bonus, you will receive the “formula” via email as a thank you for your time.