The Art and Science of Sales Forecasting: More Than Just Crystal Ball Gazing
Let’s be honest – most sales forecasting feels about as reliable as using a Magic 8-Ball to predict next quarter’s revenue. We’ve all been there: staring at spreadsheets, trying to make sense of historical data while our CEO breathes down our neck asking for “accurate projections.”
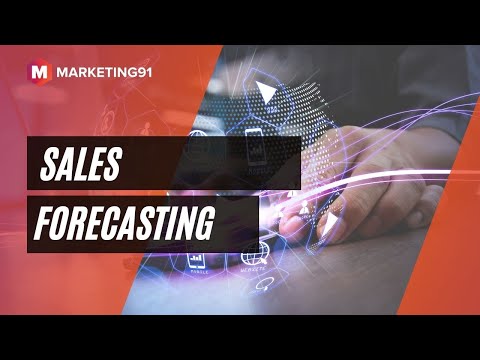
The disconnect between what we want from sales forecasting and what we actually get isn’t about a lack of tools or data. It’s about how we fundamentally approach the process. We treat it like some mystical art when it’s really more of a “choose your own adventure” story – one where every choice impacts the ending, but there’s always a logical path forward.
Why Traditional Sales Forecasting Techniques Are Failing Modern Businesses

Here’s the thing about sales forecasting that nobody wants to admit: most businesses are still using methods better suited for the 1990s than the 2020s. They’re trying to predict the future using rear-view mirror analytics in a world where market conditions change faster than a TikTok trend.
Think about it – when was the last time your sales forecast actually accounted for a viral social media post about your product? Or considered the impact of a competitor’s flash sale that wasn’t even planned when you made your projections? Traditional forecasting methods just weren’t built for this kind of dynamic market environment.
The Real Cost of Poor Forecasting
Bad forecasts aren’t just annoying – they’re expensive. Like that time I worked with an ecommerce brand that underestimated holiday demand by 40% because they didn’t factor in their influencer campaign’s viral success. The result? Stockouts during their peak selling season and about $2M in lost revenue. Ouch.
Modern Sales Forecasting Techniques: A New Approach
The good news? We’re not stuck with outdated forecasting methods. The better news? You don’t need a PhD in data science to implement better forecasting techniques. What you need is a framework that combines the best of human insight with the power of modern analytics.
The Foundation: Understanding Your Data Patterns
Before diving into complex forecasting models, you need to understand what your sales data is actually telling you. It’s like being a detective – looking for patterns, seasonality, and anomalies that might indicate deeper trends. Are your sales cyclical? Do they spike during certain events? Is there a correlation with external factors like weather or economic indicators?
Time Series Analysis: Beyond Basic Trending
Time series analysis sounds fancy, but at its core, it’s just a structured way of looking at how your sales change over time. The key is choosing the right method for your business pattern. If you’re seeing regular seasonal fluctuations, something like the Holt-Winters method might be your best bet. For more stable patterns, simpler moving averages could do the trick.
Machine Learning: Your New Forecasting Superpower
Here’s where things get interesting. Machine learning in sales forecasting isn’t about replacing human judgment – it’s about augmenting it. Think of ML as your analytical intern who never sleeps, constantly processing data to spot patterns humans might miss. The real magic happens when you combine ML insights with human expertise.
Choosing the Right Forecasting Technique for Your Business
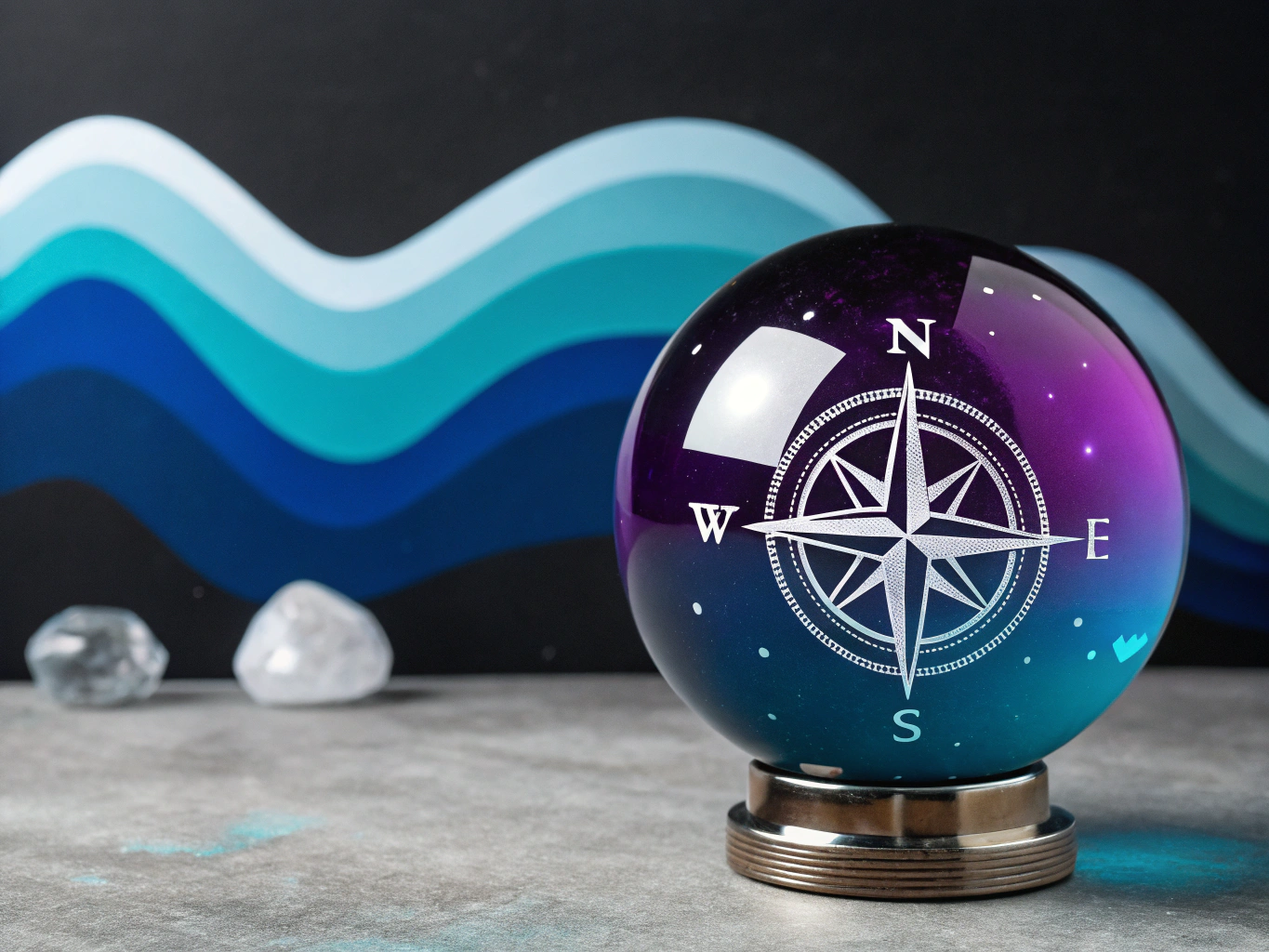
The most sophisticated forecasting model isn’t always the best choice. I’ve seen startups waste months implementing complex ML systems when a well-structured moving average would have worked just fine. The key is matching the technique to your business reality.
For Established Businesses with Stable Sales
- Traditional time series methods often work well
- Focus on identifying and accounting for seasonal patterns
- Consider multiple regression for understanding external factors
For High-Growth or Volatile Businesses
- Machine learning models can better capture complex patterns
- Hybrid approaches combining multiple methods
- More frequent forecast updates and adjustments
The reality is, most businesses need a combination of techniques. It’s like having different tools in your toolbox – sometimes you need a hammer, sometimes you need a screwdriver, and sometimes you need that weird specialized tool you bought for one specific job but turns out to be surprisingly useful.
The Human Element in Modern Forecasting
Let’s address the elephant in the room: AI and machine learning aren’t going to replace human judgment in sales forecasting. They’re going to enhance it. The best forecasts come from combining computational power with human insight – understanding not just what the numbers say, but what they mean in the context of your business reality.
I’ve seen too many companies get caught up in the allure of fully automated forecasting, only to realize that without human oversight, they’re missing crucial context. Like the time an AI model predicted a massive sales spike for a summer product line in December – technically correct based on the data, but practically nonsensical given the product’s seasonal nature.
Advanced Sales Forecasting Techniques: When AI Meets Human Intuition

Let’s be honest – most sales forecasting feels like trying to predict the weather with a magic 8-ball. We’ve all been there, staring at spreadsheets, hoping historical data will somehow perfectly map to future results. But here’s the thing: while traditional forecasting methods aren’t exactly obsolete, they’re about as cutting-edge as a flip phone in 2024.
The game has changed. We’re seeing AI and machine learning transform sales forecasting from an educated guessing game into something that actually works. Think of it like upgrading from that magic 8-ball to a sophisticated weather radar system – still not perfect, but dramatically more reliable.
The Evolution of Sales Forecasting Techniques
Remember when “gut feel” was considered a legitimate forecasting technique? (Some of you are nervously shifting in your seats right now). While intuition still plays a role, modern sales forecasting is increasingly driven by data and AI-powered analysis. It’s like having a time machine that runs on algorithms instead of plutonium.
Machine Learning Models: The New Heavy Lifters
Machine learning models are becoming the workhorses of modern sales forecasting. These aren’t your grandfather’s regression models – we’re talking about sophisticated systems that can process massive amounts of data and identify patterns humans would never spot.
- XGBoost and Random Forests: Perfect for handling complex relationships between variables
- LSTM Networks: Excellent at remembering long-term patterns in sales data
- Prophet: Facebook’s gift to time series forecasting (and yes, it’s actually good)
Hybrid Approaches: Where Human Meets Machine
Here’s where it gets interesting – and where most companies get it wrong. The most effective forecasting techniques don’t rely solely on AI or human judgment. They combine both. Think of it as a partnership where AI handles the heavy lifting of data processing, while humans provide context and sanity checks.
In fact, many companies are exploring predictive sales analysis to strike this balance effectively.
The Time Series Trifecta

When it comes to time series forecasting, three techniques stand out as particularly effective for ecommerce brands:
1. SARIMA (Seasonal ARIMA)
SARIMA is like that detail-oriented friend who remembers everything – including seasonal patterns. It’s particularly effective for businesses with strong seasonal variations, like fashion or holiday-dependent products. But let’s be real – it can be as temperamental as a cat on a hot tin roof when it comes to parameter tuning.
2. Prophet
Facebook’s Prophet is the Swiss Army knife of forecasting tools. It handles seasonality, holidays, and trend changes with surprising grace. The best part? It’s relatively forgiving when your data isn’t perfect (which, let’s face it, it never is).
3. LSTMs (Long Short-Term Memory Networks)
These neural networks are the heavy artillery of sales forecasting. They’re particularly good at capturing long-term dependencies in your data, but they’re also resource-hungry beasts that need substantial data to perform well.
Practical Implementation: Beyond the Theory
Here’s where the rubber meets the road. Implementing these techniques isn’t just about choosing the right algorithm – it’s about creating a sustainable forecasting process that actually works in the real world.
Data Requirements: Garbage In, Garbage Out
You need clean, consistent historical data – at least 2 years’ worth for most techniques. But here’s the kicker: you also need contextual data like:
- Marketing campaign schedules
- Promotional calendars
- Competitor activities
- Economic indicators
- Social media sentiment data
The Implementation Process
Start small. Seriously. I’ve seen too many companies try to boil the ocean with their first forecasting implementation. Instead:
- Begin with a single product category or market segment
- Test multiple techniques in parallel
- Measure accuracy religiously
- Iterate and refine based on results
Common Pitfalls and How to Avoid Them

Let’s talk about what not to do – because sometimes knowing what to avoid is as important as knowing what to do.
The Over-Engineering Trap
Just because you can build a complex model doesn’t mean you should. I’ve seen companies implement neural networks when simple moving averages would have done the job. Remember: complexity should serve a purpose, not just make you feel clever.
The “Set It and Forget It” Mindset
Sales forecasting isn’t a Ronco Rotisserie – you can’t just set it and forget it. Markets change, consumer behavior evolves, and your forecasting methods need to adapt. Regular monitoring and adjustment are crucial.
Looking Ahead: The Future of Sales Forecasting
The future of sales forecasting is looking pretty wild, and I mean that in the best possible way. We’re seeing the emergence of real-time forecasting capabilities, enhanced by edge computing and IoT data. Imagine having your forecasts automatically update as new data flows in – that’s not science fiction anymore, it’s happening now.
But here’s the thing that really excites me: the democratization of advanced forecasting techniques. Tools are becoming more accessible, more user-friendly, and more powerful. You don’t need a PhD in statistics to leverage machine learning for forecasting anymore (though it still helps to know what you’re doing).
Machine Learning in Sales Forecasting: The Future is Already Here
Look, I’ve spent years working with AI systems, and there’s something fascinating happening in the sales forecasting space. We’re at this weird intersection where the hype around AI meets actual, practical applications that are changing how brands predict their future.
Remember when “AI-powered forecasting” meant basic regression models with a fancy UI slapped on top? Those days are gone. Modern machine learning approaches are doing things that would’ve seemed like science fiction just a few years ago. But here’s the catch – they’re not the magical crystal balls some vendors would have you believe.
The Real Deal with ML in Sales Forecasting
Let me break this down in a way that won’t make your eyes glaze over. Think of traditional forecasting like trying to predict tomorrow’s weather by looking at today’s sky. Machine learning is more like having thousands of weather stations, satellite data, and historical patterns all working together to give you a better picture.
The game-changer isn’t just the algorithms (though they’re pretty impressive) – it’s how ML handles complexity. Traditional forecasting techniques start breaking down when you throw in too many variables. But ML? It thrives on complexity. It’s like having an intern who can somehow keep track of millions of data points without getting overwhelmed.
By using advanced sales forecasting methods, businesses can enhance their predictive accuracy significantly.
When ML Actually Makes Sense (And When It Doesn’t)
Here’s the thing most consultants won’t tell you: ML isn’t always the answer. If you’re a small brand with limited historical data, jumping straight into deep learning is like using a rocket launcher to open a jar of pickles. You might want to start with simpler techniques.
But if you’re dealing with:
- Multiple product lines with complex seasonality
- Tons of historical data gathering dust in your systems
- External factors that influence your sales (think weather, social media trends, competitor actions)
Then ML starts looking pretty attractive. We’ve seen brands at ProductScope reduce forecast errors by 30-40% just by properly implementing basic ML models.
The Human Element in Advanced Forecasting
Here’s something that might surprise you: the best forecasting systems aren’t fully automated. They’re hybrid approaches that combine machine intelligence with human insight. It’s like having a really smart assistant who can crunch numbers faster than humanly possible, but still needs your judgment for the final call.
Making It Work in the Real World
I’ve seen too many companies get caught up in the technical aspects while forgetting the basics. Your fancy ML model is only as good as the data you feed it and the people interpreting its outputs. Some practical tips that actually work:
- Start small – pick one product line or category for your ML pilot
- Clean your data before you do anything else (garbage in = garbage out)
- Build in feedback loops where humans can override the system when needed
- Document everything – what works, what doesn’t, and why
Looking Ahead: The Future of Sales Forecasting
We’re entering an era where AI and ML aren’t just buzzwords in sales forecasting – they’re becoming fundamental tools. But here’s what’s really exciting: the democratization of these technologies. What used to require a team of data scientists can now be done with user-friendly tools and platforms.
The future isn’t about replacing human judgment – it’s about augmenting it. Imagine having a system that not only predicts sales but explains its reasoning, learns from its mistakes, and adapts to changing market conditions in real-time. We’re not quite there yet, but we’re getting closer every day.
Final Thoughts
Sales forecasting isn’t just about predicting numbers – it’s about making better business decisions. Whether you’re using simple moving averages or sophisticated neural networks, the goal remains the same: reducing uncertainty and improving outcomes.
The best approach? Stay practical but forward-thinking. Don’t get caught up in the AI hype, but don’t dismiss its potential either. Start with what you need, not what’s trendy. And remember, even the most sophisticated AI is still just a tool – albeit a pretty impressive one – in your business toolkit.
For ecommerce brands and content creators, the message is clear: the future of sales forecasting is neither purely human nor purely machine. It’s finding the right balance between both, using technology to enhance rather than replace human judgment. And that’s something worth getting excited about.
👉👉 Create Photos, Videos & Optimized Content in minutes 👈👈
Related Articles:
- Sales Forecasting Mastery: Predict Growth With Precision
- How Much Does the Average Shopify Store Make per Month?
- The Complete Guide to Helium 10 Freedom Ticket – ProductScope AI
- Amazon Review Analysis Tool Comparison & Buyer Guide
- ASIN: The Essential Amazon Product ID Number Explained
Frequently Asked Questions
What is the best forecasting model for sales?
The best forecasting model for sales often depends on the specific needs and circumstances of a business, but many companies find success using time series analysis or machine learning models. Time series models like ARIMA can effectively capture patterns over time for businesses with stable historical data. Machine learning models, such as regression trees or neural networks, are powerful for handling complex and non-linear data but require more sophisticated resources and expertise.
What is a sales forecasting tool?
A sales forecasting tool is software designed to help businesses predict future sales revenue based on historical data and market analysis. These tools often incorporate various forecasting methods and algorithms, providing businesses with insights to make informed decisions about inventory management, staffing, and budgeting. Examples include Salesforce, HubSpot, and Zoho CRM, which offer features tailored to different industry needs.
What are the 5 steps of the sales forecasting process?
The five steps of the sales forecasting process include: 1) Collecting and analyzing historical sales data to establish a baseline; 2) Identifying market trends and external factors that could influence future sales; 3) Selecting the appropriate forecasting method or model; 4) Applying the chosen method to predict future sales; and 5) Reviewing and refining the forecast by comparing it against actual outcomes and adjusting models as necessary.
What are the four types of forecasting?
The four types of forecasting are qualitative forecasting, quantitative forecasting, causal forecasting, and time series forecasting. Qualitative forecasting relies on expert opinions and market research, making it useful when historical data is limited. Quantitative forecasting uses mathematical models and historical data to predict future outcomes. Causal forecasting examines relationships between variables, while time series forecasting analyzes patterns within historical data over time.
What is a sales forecasting method?
A sales forecasting method is a systematic approach used to predict future sales revenue based on historical data, market trends, and other influencing factors. Methods can be qualitative, such as expert judgment or market research, or quantitative, like statistical models and machine learning algorithms. Each method has its strengths and is chosen based on the business’s specific needs and data availability.
About the Author
Vijay Jacob is the founder and chief contributing writer for ProductScope AI focused on storytelling in AI and tech. You can follow him on X and LinkedIn, and ProductScope AI on X and on LinkedIn.
We’re also building a powerful AI Studio for Brands & Creators to sell smarter and faster with AI. With PS Studio you can generate AI Images, AI Videos, Blog Post Generator and Automate repeat writing with AI Agents that can produce content in your voice and tone all in one place. If you sell on Amazon you can even optimize your Amazon Product Listings or get unique customer insights with PS Optimize.
🎁 Limited time Bonus: I put together an exclusive welcome gift called the “Formula,” which includes all of my free checklists (from SEO to Image Design to content creation at scale), including the top AI agents, and ways to scale your brand & content strategy today. Sign up free to get 200 PS Studio credits on us, and as a bonus, you will receive the “formula” via email as a thank you for your time.