Remember when we thought spreadsheets were cutting-edge tech for predicting sales? Those were simpler times. Now we’re swimming in data, wrestling with AI models, and trying to figure out if that spike in sales was because of our killer marketing campaign or just because Taylor Swift wore something similar on Instagram.
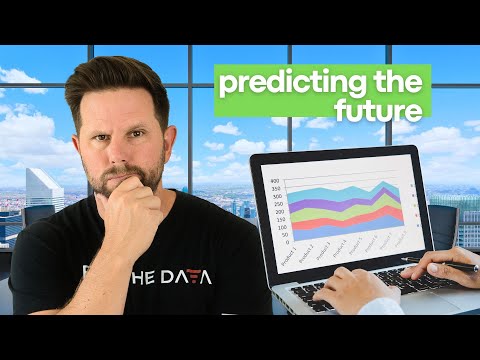
Here’s the thing about sales forecasting that nobody talks about: we’ve overcomplicated it while simultaneously oversimplifying it. We’re either stuck in Excel hell with basic moving averages, or we’re throwing neural networks at the problem hoping they’ll magically solve everything. Neither approach is quite right.
Why Traditional Sales Forecasting Methods Are Failing Us
Let’s be real – most businesses are still using forecasting methods that were developed when bell-bottoms were in fashion (the first time around). These methods worked fine when the biggest variable was seasonal demand. But in our hyper-connected, social-media-driven marketplace? They’re about as useful as a paper map in the age of GPS.
The problem isn’t just outdated methods – it’s our entire approach to sales forecasting. We’re trying to predict the future using tools designed for a world that no longer exists. Think about it: how can a simple trend line account for a TikTok video going viral and creating instant demand for your product?
The Evolution of Sales Forecasting: From Guesswork to Data Science

The Old Way: Intuition-Based Forecasting
Remember when sales forecasting was basically your most experienced sales rep saying “Yeah, I think we can do about 20% better than last year”? While there’s still value in human intuition, relying solely on gut feelings is like trying to navigate a spacecraft with a compass.
The Current Reality: Data-Driven but Disconnected
Most companies now use some form of data-driven forecasting, but they’re missing the bigger picture. They’re collecting mountains of data but struggling to turn it into actionable insights. It’s like having all the ingredients for a gourmet meal but following a recipe for microwave dinner.
Understanding Modern Sales Forecasting Methods
Before we dive into the cutting-edge stuff, let’s break down the core methods that still form the foundation of sales forecasting. Think of these as your basic cooking techniques – you need to master them before you can start experimenting with molecular gastronomy.
Statistical Methods: The Workhorses of Forecasting
Moving averages, exponential smoothing, and regression analysis – these are your bread and butter. They’re not sexy, but they’re reliable when used correctly. The key is knowing their limitations. Moving averages are great for stable markets but fall apart when there’s sudden change (hello, pandemic economy).
Time Series Analysis: Pattern Recognition on Steroids
This is where we start getting more sophisticated. Time series analysis looks at your sales data as a complex pattern of trends, seasonal variations, and random fluctuations. It’s like being able to read the weather patterns of your business – useful, but still not perfect in predicting those unexpected storms.
The AI Revolution in Sales Forecasting

Now we’re entering my favorite territory – where artificial intelligence meets sales forecasting. But let’s be clear: AI isn’t a magic wand. It’s more like having a really smart intern who can process vast amounts of data but still needs guidance and oversight.
Machine Learning Models: Beyond Simple Predictions
Modern ML models can handle multiple variables simultaneously, learning from patterns that would be impossible for humans to spot. They can incorporate everything from social media sentiment to weather patterns to competitor pricing. But – and this is a big but – they’re only as good as the data they’re fed and the humans training them.
Deep Learning Applications in Forecasting
Neural networks, particularly those designed for time series prediction, are becoming increasingly powerful. They can capture complex non-linear relationships and adapt to changing market conditions. But they require massive amounts of clean, reliable data – something many businesses still struggle with.
The Human Element in Modern Forecasting
Here’s something that might surprise you: as our forecasting tools become more sophisticated, the human element becomes more important, not less. AI can crunch numbers and spot patterns, but it can’t understand market psychology or predict how a new product will make customers feel.
Combining Artificial and Human Intelligence
The most effective forecasting systems I’ve seen combine machine learning capabilities with human insight. It’s like having a chess computer and a grandmaster working together – each brings something unique to the table. The trick is knowing when to trust the algorithm and when to trust your gut.
And this brings us to a crucial point: successful sales forecasting isn’t just about choosing the right method – it’s about creating a forecasting ecosystem that can evolve with your business. It needs to be flexible enough to incorporate new data sources, robust enough to handle market disruptions, and simple enough that your team will actually use it.
Advanced Statistical Forecasting Techniques

Let’s be honest – traditional forecasting methods can feel like trying to predict the weather by looking at clouds. Sure, you might get lucky sometimes, but you’re probably going to get caught in the rain without an umbrella.
That’s where advanced statistical methods come in. They’re like having a super-powered weather radar in your pocket. But here’s the thing – they’re not magic. They’re tools, and like any tool, knowing when and how to use them makes all the difference.
The Evolution of Statistical Forecasting
Remember when Excel spreadsheets were considered cutting-edge? Those days are long gone. Today’s statistical forecasting methods are like Excel on steroids – they can handle multiple variables, recognize complex patterns, and even learn from their mistakes. But they still need human oversight to make sense of it all.
Think of it this way: if traditional forecasting is like trying to navigate with a paper map, modern statistical methods are like having GPS. Both can get you there, but one’s a lot more precise and can adjust in real-time when conditions change.
Exponential Smoothing: Not Your Grandfather’s Moving Average
Exponential smoothing is the Swiss Army knife of forecasting tools. It’s versatile, reliable, and surprisingly powerful when used correctly. The beauty of exponential smoothing lies in its ability to give more weight to recent data while still considering historical patterns – kind of like how you probably care more about last month’s sales than what happened two years ago.
Here’s where it gets interesting: there are different flavors of exponential smoothing, each designed for specific scenarios. Simple exponential smoothing works great for stable patterns, while Holt-Winters can handle both trends and seasonality. It’s like having different settings on your camera – each one’s optimized for specific conditions.
Regression Analysis: Finding the Story Behind the Numbers
You know how sometimes you can just feel that certain factors are affecting your sales, but you can’t quite put your finger on it? That’s where regression analysis shines. It’s like having a detective that can sift through mountains of data to find meaningful connections.
Multiple regression takes this even further by considering several factors simultaneously. Want to know how weather patterns, social media sentiment, and competitor pricing all impact your sales? Multiple regression can help connect those dots. Just remember – correlation doesn’t always mean causation. I’ve seen too many brands chase false patterns because they didn’t validate their assumptions.
Machine Learning and AI-Driven Sales Forecasting
Now we’re getting to the good stuff. Machine learning in sales forecasting is like having an intern who never sleeps, never complains, and gets smarter every day. But just like with any intern, you need to train them right and check their work.
The Rise of Neural Networks
Neural networks are fascinating beasts. They’re designed to mimic how our brains process information, but they can analyze patterns at a scale no human could match. I’ve seen neural networks predict seasonal trends that even experienced sales managers missed – patterns so subtle they were invisible to the naked eye.
But here’s the catch: neural networks are data hungry. They need lots of clean, reliable data to perform well. It’s like trying to teach someone a language – the more examples they have, the better they’ll learn.
Gradient Boosting: The New Kid on the Block
Gradient boosting models have been crushing it in forecasting competitions lately. They’re particularly good at handling the messy, real-world data that most ecommerce brands deal with. Think of them as ensemble performers – each model builds on the strengths of the previous ones, learning from their mistakes.
XGBoost, LightGBM, and CatBoost are the current stars of the show. They’re like different martial arts styles – each has its strengths, and choosing the right one depends on your specific needs and constraints.
Deep Learning: When Simple Just Won’t Cut It
Deep learning is where things get really interesting. These models can handle incredibly complex patterns and relationships. They’re particularly good at dealing with what we call “non-linear” relationships – when the connection between cause and effect isn’t straightforward.
But let’s be real: deep learning isn’t always the answer. Sometimes it’s like using a sledgehammer to hang a picture – overkill for simple forecasting needs. The key is knowing when to deploy these powerful tools and when to stick with simpler methods.
Hybrid Approaches: The Best of Both Worlds

Here’s where the magic really happens. Hybrid approaches combine different forecasting methods to create something greater than the sum of its parts. It’s like having a team of specialists instead of trying to find one person who can do everything.
Combining Statistical and ML Methods
I love hybrid approaches because they’re pragmatic. They acknowledge that different methods have different strengths. Maybe you use traditional statistical methods for your baseline forecast, then layer on machine learning to catch subtle patterns and anomalies. It’s about using the right tool for each part of the job.
The trick is in the integration. You need clear rules for when to use each method and how to reconcile different forecasts. Think of it like cooking – knowing your ingredients is important, but knowing how to combine them is what makes a great dish.
The Role of Human Judgment
Let’s not forget the human element. The best forecasting systems I’ve seen combine sophisticated algorithms with human expertise. AI can crunch numbers faster than any human, but it can’t understand context like we do. It won’t know about that upcoming influencer collaboration or that competitor who’s about to launch a similar product.
That’s why I always advocate for what I call “augmented forecasting” – using AI to enhance human decision-making, not replace it. It’s about finding that sweet spot where technology and human insight work together seamlessly.
Measuring and Improving Forecast Accuracy
Look, we’ve all been there – staring at a forecast that’s way off and wondering where things went sideways. The truth is, even the fanciest AI models can miss the mark if we’re not measuring and tweaking them properly. Let’s break down how to actually make these predictions work in the real world.
The Metrics That Actually Matter
MAPE, RMSE, MAE… the alphabet soup of forecast accuracy metrics can make your head spin. But here’s what you really need to know: MAPE (Mean Absolute Percentage Error) is your bread and butter for comparing accuracy across different product lines. It’s like getting a percentage score on a test – straightforward and actionable.
But here’s the catch that most forecasting tools won’t tell you: MAPE can be misleading when your sales numbers are close to zero. I’ve seen ecommerce brands torture themselves over massive percentage errors on products that only sell a few units per month. In these cases, you’re better off using plain old Mean Absolute Error (MAE).
The Art of Error Analysis
Think of forecast errors like breadcrumbs leading you back to what went wrong. Are you consistently overshooting during holidays? Underestimating the impact of social media campaigns? These patterns are gold mines for improvement – if you know where to look.
I once worked with a brand that couldn’t figure out why their forecasts were always off in July. Turns out, they were missing a crucial pattern: their Instagram influencer posts consistently drove 3x normal sales volume during mid-summer. The data was trying to tell them a story, but they weren’t listening.
Future Trends in Sales Forecasting
Let’s get real about where this is all heading. The future isn’t about AI replacing human judgment – it’s about AI becoming that super-smart intern who does the heavy lifting while we focus on strategy.
The Rise of Hybrid Intelligence
We’re seeing a fascinating shift toward what I call “hybrid intelligence” in forecasting. Imagine combining the pattern-recognition capabilities of machine learning with the intuitive understanding of seasoned sales managers. It’s not about choosing between human or machine – it’s about finding the sweet spot where both shine.
For example, ProductScope AI’s forecasting tools don’t just crunch numbers – they learn from how successful brands adjust their forecasts manually, creating a feedback loop that gets smarter over time. Think of it as AI with street smarts.
Real-Time Adaptability
The days of monthly forecast updates are becoming as outdated as dial-up internet. Modern forecasting systems are moving toward continuous adjustment – like a GPS recalculating your route in real-time when traffic patterns change.
But here’s the thing about real-time forecasting that nobody talks about: it can drive you crazy if you let it. The key is setting appropriate thresholds for when to actually act on these updates. Not every wiggle in your data deserves a response.
Conclusion: Making It All Work
After diving deep into the world of sales forecasting methods, here’s what I want you to take away: the best forecast isn’t necessarily the most complex one. It’s the one that helps you make better decisions consistently.
Start simple. Get the basics right. Then gradually add sophistication as your needs (and capabilities) grow. Remember, Amazon didn’t build their predictive powerhouse overnight – they started with basic time series analysis and built from there.
Action Steps for Better Forecasting
- Audit your current forecasting accuracy – you can’t improve what you don’t measure
- Pick one area where better forecasting would immediately impact your bottom line
- Test a new method or tool in a controlled way – maybe with a single product category
- Build a feedback loop to capture what works and what doesn’t
The future of sales forecasting isn’t about finding a crystal ball – it’s about building a system that helps you make smarter decisions with incomplete information. And isn’t that what business is all about?
Remember: the goal isn’t perfect predictions. It’s better decisions. Keep that in mind, and you’ll be ahead of 90% of your competition who are still chasing forecast accuracy decimals while missing the bigger picture.
Now go forth and forecast – but maybe start with those high-margin products first. Just saying.
👉👉 Create Photos, Videos & Optimized Content in minutes 👈👈
Related Articles:
- Sales Forecasting Mastery: Predict Growth With Precision
- How Much Does the Average Shopify Store Make per Month?
- Optimize Your Stock: Best Inventory Management Tools for Amazon
Frequently Asked Questions
What is the best forecasting model for sales?
The best forecasting model for sales often depends on the specific context and needs of the business. However, time series models like ARIMA and exponential smoothing are commonly used due to their ability to handle historical sales data effectively. Machine learning models such as regression trees and neural networks are also gaining popularity for their predictive accuracy and ability to handle large datasets. Ultimately, the choice of model should consider factors like data availability, forecast horizon, and the complexity of the sales environment.
What is a sales forecasting tool?
A sales forecasting tool is a software application designed to help businesses predict future sales performance based on historical data and various analytical techniques. These tools often include features like data visualization, trend analysis, and scenario planning to provide insights into potential sales outcomes. By automating data collection and analysis, sales forecasting tools enable businesses to make informed decisions, allocate resources efficiently, and develop strategic plans with greater confidence.
What are the 5 steps of the sales forecasting process?
The 5 steps of the sales forecasting process typically include: 1) Data Collection, where relevant historical sales data is gathered; 2) Data Analysis, involving the examination of trends and patterns in the collected data; 3) Selection of a Forecasting Method, choosing an appropriate model based on the analysis; 4) Forecasting, using the selected method to predict future sales; and 5) Monitoring and Adjustment, regularly reviewing forecasts against actual sales to refine and improve accuracy over time.
What are the four types of forecasting?
The four types of forecasting generally include qualitative forecasting, quantitative forecasting, time series forecasting, and causal forecasting. Qualitative forecasting relies on expert opinions and market research, while quantitative forecasting uses numerical data and statistical methods. Time series forecasting focuses on identifying patterns over time in historical data, whereas causal forecasting examines the relationships between different variables to predict future trends. Each type has its own applications and is chosen based on data availability and the nature of the forecast needed.
What is a sales forecasting method?
A sales forecasting method is a systematic approach used to predict future sales based on historical data, market analysis, and statistical techniques. These methods can range from simple techniques like moving averages to more complex models such as econometric modeling or machine learning algorithms. The choice of method often depends on the specific needs of the business, the availability of data, and the desired accuracy and granularity of the forecast.
About the Author
Vijay Jacob is the founder and chief contributing writer for ProductScope AI focused on storytelling in AI and tech. You can follow him on X and LinkedIn, and ProductScope AI on X and on LinkedIn.
We’re also building a powerful AI Studio for Brands & Creators to sell smarter and faster with AI. With PS Studio you can generate AI Images, AI Videos, Blog Post Generator and Automate repeat writing with AI Agents that can produce content in your voice and tone all in one place. If you sell on Amazon you can even optimize your Amazon Product Listings or get unique customer insights with PS Optimize.
🎁 Limited time Bonus: I put together an exclusive welcome gift called the “Formula,” which includes all of my free checklists (from SEO to Image Design to content creation at scale), including the top AI agents, and ways to scale your brand & content strategy today. Sign up free to get 200 PS Studio credits on us, and as a bonus, you will receive the “formula” via email as a thank you for your time.