The Art and Science of Review Analysis: More Than Just Stars and Comments
Remember when we used to walk into stores and just… buy stuff? No checking reviews, no scrolling through endless comments, no analysis paralysis. Those days are long gone—and honestly, good riddance. But here’s the thing: while we’re drowning in reviews, most brands and creators are barely scratching the surface of what this goldmine of customer feedback can really tell us.
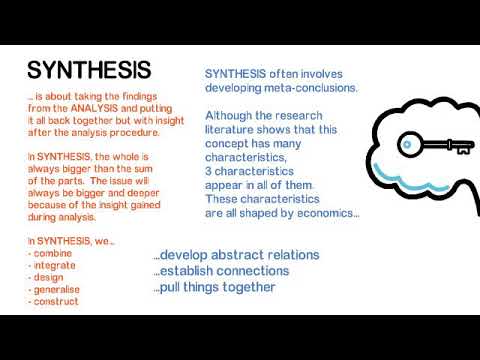
I’ve spent years helping ecommerce brands decode their customer feedback, and I’ll tell you this: review analysis isn’t just about counting stars or tracking sentiment. It’s about understanding the story behind the data—the human narrative that emerges when you know where to look.
Understanding Review Analysis: Beyond the Basics
Let’s get something straight: review analysis isn’t just tallying up 5-star ratings or scanning for keywords like “great” or “terrible.” That’s like trying to understand a person by only looking at their emoji usage. The real magic happens when you start seeing reviews as conversations—multilayered dialogues between customers and brands that reveal deeper truths about products, experiences, and expectations.
The Evolution of Review Intelligence
Back in the early days of ecommerce, review analysis was pretty primitive. We’d basically count positive versus negative reviews and call it a day. But just like how AI has evolved from being a clunky chatbot to something that can write poetry (albeit sometimes questionable poetry), review analysis has transformed into something far more sophisticated.
Today’s review analysis is like having a thousand focus groups running 24/7, except instead of being in a stuffy room with a two-way mirror, your customers are sharing their unfiltered thoughts from their couch, probably in their pajamas. And that’s exactly where the gold is—in that unstructured, honest feedback.
The Psychology Behind Reviews
Here’s something fascinating: people don’t just write reviews to help others make buying decisions. They write reviews to be part of a community, to express their identity, to feel heard. I’ve seen countless reviews where people write entire stories about their experience with a product, complete with character development and plot twists. These aren’t just reviews—they’re micro-narratives that tell us as much about the reviewer as they do about the product.
Breaking Down the Review Analysis Process
Think of review analysis like archaeology for the digital age. Instead of digging through dirt looking for artifacts, we’re sifting through data looking for patterns. But just like archaeology, you need the right tools and methods to avoid destroying valuable information in the process.
The Three Pillars of Modern Review Analysis
1. Sentiment Analysis: This goes way beyond positive/negative classification. Modern sentiment analysis can detect nuances like sarcasm, enthusiasm, disappointment, and even brand loyalty. It’s like having an emotional radar that can pick up the subtlest signals in customer feedback.
2. Feature Extraction: This is where AI really shines. Instead of manually tagging mentions of product features, AI can automatically identify and categorize what customers are talking about. It’s like having a super-powered assistant who can read thousands of reviews and tell you exactly what features people love, hate, or wish you’d add.
3. Trend Analysis: This is about connecting the dots across time and space. Are complaints about a specific issue increasing? Do positive reviews spike after certain changes? It’s like having a time machine that lets you see how customer sentiment evolves.
The Truth About Amazon Reviews
Let’s talk about the elephant in the room: Amazon reviews. Yes, fake reviews are a thing. Yes, they’re a problem. But here’s what most people don’t realize: sophisticated review analysis can actually help identify fake reviews with surprising accuracy. It’s not just about looking for suspicious patterns—it’s about understanding the linguistic fingerprints that genuine reviews tend to leave.
I’ve seen brands waste thousands of dollars trying to combat fake reviews with basic tools, when what they really needed was a more nuanced approach to understanding their review ecosystem. The key isn’t just filtering out the fake stuff—it’s amplifying the genuine signals in your review data.
Real Reviews vs. Manufactured Feedback
Here’s a truth bomb: some of the most valuable reviews aren’t the perfectly written 5-star essays. They’re the messy, emotional, typo-filled reactions from real customers who were moved enough by their experience to share it. These raw, unfiltered reviews often contain the most actionable insights for brands looking to improve.
The Science Behind Review Analysis
Let’s get real for a moment – we’re drowning in reviews. Every product, service, and random thought gets rated these days. But here’s what fascinates me: behind all those stars and comments lies a goldmine of psychological patterns that would make Freud’s head spin.
The Psychology of Reviews: Why We Write What We Write
Think about the last time you left a review. Was it because you were delighted? Furious? There’s rarely an in-between, right? That’s because we humans are wonderfully irrational creatures when it comes to feedback. We’re more likely to write a review when we’re experiencing extreme emotions – it’s like our brains have an urgent need to broadcast our joy or disappointment to the world.
But here’s where it gets interesting: negative reviews often contain more detailed information than positive ones. It’s like our brains go into detective mode when we’re upset, documenting every single thing that went wrong. Positive reviews? Usually more vague, more emotional. “Great product!” isn’t exactly giving us the deep insights we’re looking for.
Natural Language Processing: Teaching Machines to Read Between the Lines
Remember that intern analogy I love using for AI? Well, in review analysis, we’ve basically got millions of interns reading through comments at lightning speed. Natural Language Processing (NLP) is the technology that makes this possible, and it’s getting scary good at understanding human nuance.
Think of NLP as your most detail-oriented team member who never gets tired of reading reviews. It breaks down text into meaningful chunks, identifies sentiment, and spots patterns that human analysts might miss. But here’s the kicker – it’s not just looking for obvious stuff like “good” or “bad.” Modern NLP can pick up on sarcasm, implied meaning, and even cultural context.
The Statistical Symphony of Sentiment
Numbers don’t lie, but they sure can dance. When we’re doing review analysis at ProductScope AI, we’re not just counting stars – we’re orchestrating a complex statistical ballet that reveals the true story behind customer feedback.
Here’s what most people get wrong about sentiment analysis: they think it’s just about sorting reviews into positive and negative buckets. But real sentiment analysis is more like reading the emotional temperature of your entire customer base across multiple dimensions. Are they frustrated with shipping times but love the product quality? Are they raving about customer service but concerned about pricing?
From Data to Decisions: Making Review Analysis Actionable
All this fancy analysis means nothing if you can’t turn it into actual improvements. I’ve seen too many brands collect mountains of review data only to let it gather digital dust. The real magic happens when you create feedback loops that turn customer insights into product evolution.
Review Collection Strategies That Actually Work
First things first – you need to get those reviews flowing. But here’s the thing: asking for reviews is an art form. Timing is everything. Send that request too early, and customers haven’t had time to form an opinion. Too late, and the experience is no longer fresh in their minds.
Pro tip: the sweet spot for product reviews? Usually 7-10 days after delivery for physical products, or after the second or third use for digital products. And please, for the love of all things tech, don’t offer incentives for positive reviews. Not only is it ethically questionable, but it also skews your data and can get you in hot water with platforms like Amazon.
Tools of the Trade: Beyond Basic Analytics
The tools landscape for review analysis is like the Wild West right now – lots of options, not all of them good. You’ve got your enterprise-level platforms that cost more than a startup’s annual budget, and then you’ve got scrappy open-source solutions that require a PhD in computer science to operate.
At ProductScope AI, we’ve found that the best approach is usually a hybrid one. Combine automated tools for the heavy lifting (sentiment analysis, trend spotting, competitor monitoring) with human insight for the nuanced stuff. It’s like having a really smart calculator – it’ll crunch the numbers, but you need human wisdom to interpret what those numbers mean for your business.
Turning Insights into Action
Here’s where most brands drop the ball – they collect all this amazing feedback and then… nothing happens. Review analysis should be a living, breathing part of your product development cycle. Every major feature update, every UI change, every customer service policy should be informed by what your reviews are telling you.
But don’t just react to problems – look for opportunities. Sometimes your customers are telling you what they want before they even know they want it. Those seemingly random comments about “I wish it could…” or “It would be cool if…” – that’s your product roadmap writing itself.
The real power of review analysis isn’t in the technology or the methodology – it’s in how you use it to create better experiences for your customers. Because at the end of the day, all those stars and comments are really just people trying to tell you how to serve them better.
Implementing Review Analysis at Scale
Let’s face it – most brands are drowning in review data while starving for insights. They’ve got thousands of reviews across dozens of platforms, but they’re still making product decisions based on gut feelings and scattered feedback. It’s like having a library full of books but no way to find the chapter you need.
The real magic happens when you can turn that mountain of reviews into actionable intelligence. And no, I’m not talking about basic sentiment analysis that tells you people either love or hate your product (shocker). I’m talking about the kind of deep analysis that reveals why your customers are choosing your competitors, what features they’re actually willing to pay more for, and which product improvements will drive the highest ROI.
Building Your Review Analysis Framework
Think of review analysis like training an AI model – garbage in, garbage out. You need clean, structured data to get meaningful insights. Here’s where most brands go wrong: they try to analyze everything at once instead of starting with a focused hypothesis.
Start with your most pressing business question. Are customers complaining about your new feature? Is there a specific competitor eating into your market share? Let that guide your initial analysis framework.
The Truth About Sentiment Analysis Tools
Here’s something that might ruffle some feathers: most sentiment analysis tools are about as useful as a chocolate teapot. They’ll tell you if a review is positive or negative, but miss the nuanced feedback that actually matters for product development.
What you really need is a hybrid approach that combines AI-powered analysis with human insight. At ProductScope AI, we’ve seen brands waste months trying to fully automate their review analysis, only to realize that the most valuable insights come from combining machine learning with human expertise.
Advanced Review Analysis Techniques
Want to level up your review analysis game? Here are some techniques that go beyond basic sentiment scoring:
- Feature correlation mapping: Identify which product features consistently appear together in positive reviews
- Competitive intelligence extraction: Track how customers compare your products to competitors
- Price sensitivity analysis: Understand what features justify premium pricing in customers’ minds
- Purchase intent signals: Identify language patterns that indicate high purchase probability
Separating Signal from Noise
Not all reviews are created equal. Some of your most valuable insights might come from those detailed 3-star reviews rather than the 5-star love letters or 1-star rants. The trick is knowing how to weight different types of feedback.
I’ve seen brands completely revamp their product roadmap based on insights from just a handful of detailed, thoughtful reviews. It’s not about the quantity – it’s about finding those golden nuggets of feedback that point to real market opportunities.
Making Review Analysis Actionable
Analysis without action is just intellectual entertainment. The real value comes from turning insights into improvements. Here’s your action plan:
- Create a feedback loop between your review analysis and product development team
- Set up automated alerts for emerging issues or opportunities
- Develop a scoring system for prioritizing review-based improvements
- Track the impact of changes on future review sentiment
The Future of Review Analysis
We’re entering an era where AI can help us understand not just what customers are saying, but what they really mean. Imagine being able to predict product issues before they show up in reviews, or automatically identifying emerging market trends across thousands of customer conversations.
But here’s the thing – AI isn’t going to replace human judgment in review analysis. It’s going to augment it. The most successful brands will be those that use AI to scale their analysis while keeping humans in the loop for strategic interpretation.
Final Thoughts
Review analysis isn’t just about damage control or vanity metrics. It’s about building a continuous feedback loop that drives product innovation and customer satisfaction. The brands that get this right will have an unfair advantage in understanding and serving their customers.
Remember: reviews are your customers telling you exactly what they want. Are you really listening? And more importantly, are you turning those insights into action? The tools and techniques are there. The data is there. The question is: are you ready to move beyond basic feedback and start using review analysis as a strategic advantage?
👉👉 Create Photos, Videos & Optimized Content in minutes 👈👈
Related Articles:
- Amazon Analytics Tools for Sellers – ProductScope
- Sentiment Analysis Tool Review: Top 3 Options for 2024 …
- Financial Analysis: How AI Tools Transform Investment Decisions …
Frequently Asked Questions
How to write an analysis review?
To write an analysis review, start by thoroughly reading the material you are analyzing, whether it’s a book, article, or another type of content. Identify the main themes, arguments, and evidence presented. Provide a critical evaluation by discussing the strengths and weaknesses, and offer your own insights or interpretations supported by evidence. Conclude with a summary of your findings and their implications.
What is online review analysis?
Online review analysis involves examining customer feedback left on digital platforms to extract meaningful insights about a product, service, or brand. This process often uses text analytics tools to identify common themes, sentiment, and customer satisfaction levels. It helps businesses understand consumer perceptions and make informed decisions to improve their offerings.
What are the 5 steps of analysis?
The 5 steps of analysis typically include: 1) Defining the scope and objectives of the analysis, 2) Collecting relevant data or information, 3) Organizing and processing the data to identify patterns or trends, 4) Interpreting the findings to draw conclusions, and 5) Reporting the results in a clear and concise manner, often with actionable recommendations.
What is an analytics review?
An analytics review is an evaluation of data-driven insights derived from analytical tools and techniques. It involves assessing the effectiveness of analytics processes and the accuracy of the data collected. The review aims to ensure that the insights align with business objectives and provide value for strategic decision-making.
How to do review analysis?
To conduct review analysis, start by gathering a substantial number of reviews relevant to your focus area. Use software tools to process and analyze the text for sentiment, frequency of keywords, and overall themes. Interpret the results to understand customer sentiment and identify areas for improvement or opportunities for enhancement. This process helps in tailoring strategies to better meet customer needs.
About the Author
Vijay Jacob is the founder and chief contributing writer for ProductScope AI focused on storytelling in AI and tech. You can follow him on X and LinkedIn, and ProductScope AI on X and on LinkedIn.
We’re also building a powerful AI Studio for Brands & Creators to sell smarter and faster with AI. With PS Studio you can generate AI Images, AI Videos, Blog Post Generator and Automate repeat writing with AI Agents that can produce content in your voice and tone all in one place. If you sell on Amazon you can even optimize your Amazon Product Listings or get unique customer insights with PS Optimize.
🎁 Limited time Bonus: I put together an exclusive welcome gift called the “Formula,” which includes all of my free checklists (from SEO to Image Design to content creation at scale), including the top AI agents, and ways to scale your brand & content strategy today. Sign up free to get 200 PS Studio credits on us, and as a bonus, you will receive the “formula” via email as a thank you for your time.